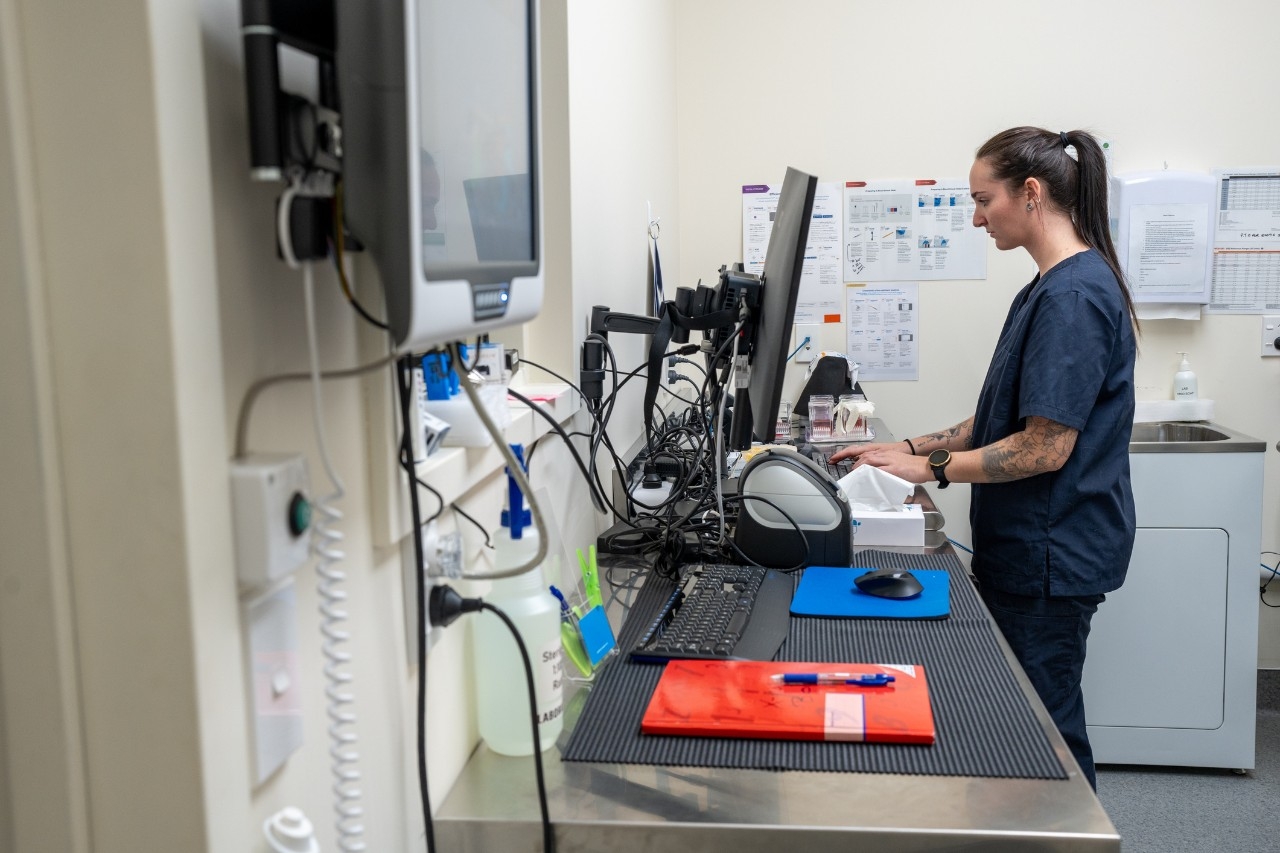
An NYU study explores AI’s potential to assist health professionals with diagnosing diseases and recommending medicines where resources are scarce
ChatGPT has shown promise in summarizing patient records, making diagnoses for certain diseases, and providing initial treatment recommendations. And the use of AI “doctors” has also begun to support mental health care. But much more testing and refinement is needed before the medical community could consider rolling it out at a large scale, particularly in under-resourced countries where some researchers believe its use could close gaps in access to the diagnosis and treatment of common diseases.
NYU data scientist Ruopeng An is a leading researcher on how AI could address public health disparities, and he and colleagues recently put ChatGPT-as-doctor through a battery of tests. Feeding it with straight-forward input from a series of mock patients, they set out to determine whether it could detect diabetes, hypertension, and tuberculosis and other illnesses accurately, and if it could suggest appropriate meds or not.
In a recent Journal of Medical Internet Research study, An and research collaborators found ChatGPT has promise as a way to provide a doctor’s or mental health counselor’s patient with an initial diagnosis. In the experiment, ChatGPT showed a strong success rate, providing appropriate medical recommendations in a majority of the instances when a patient’s symptoms were inputted.
The testing was repeated for nine different disease types in all, a total of 27 patient/Chatbot interactions. But one caveat was notable: even when it offered up a correct diagnosis, ChatGPT recommended medications that were unnecessary or potentially harmful. This happened in more than half the cases.
An, who studies AI’s potential to improve public access to advice and care in places of the world where treatment is hard to get, said more work clearly needs to be done. Until then, AI’s dependability in important areas of health care are likely to remain uncertain.
An’s growing body of work on the future uses for AI to reduce social disparities has, in part, made him a leading expert in the epidemiology of obesity. His previous study explored the potential of machine learning and deep neural networks to predict and assess a patient’s obesity progression from short audio recordings. As a faculty member at NYU’s Silver School of Social Work, he holds a Martin Silver Endowed Professorship in Data Science and Prevention and directs the Constance and Martin Silver Center on Data Science and Social Equity.
“The future,” he says, “holds exciting possibilities, with AI improving access to care, especially in underserved regions, enhancing accuracy in diagnostics, and supporting overburdened healthcare providers by automating routine tasks. Yet for this transformation to be truly revolutionary, we must address challenges around accuracy, safety, and ethical use, especially in low-resource settings.”
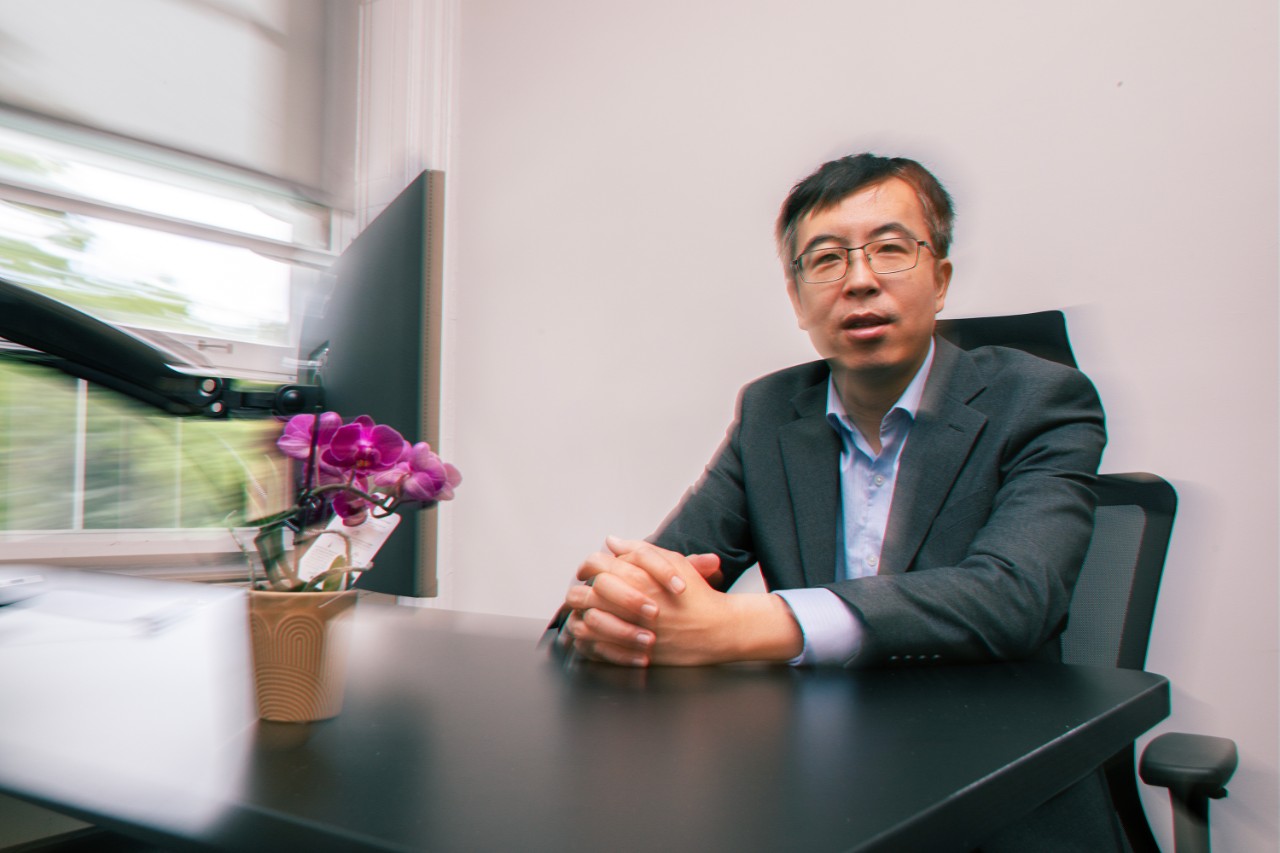
NYU News talked with him about what his latest study shows about ChatGPT’s potential, and its pitfalls.
There’s so much excitement about AI in health care. Can you break down your new study for us? How did you simulate a doctor-patient interaction, and how did Chat GPT do?
We used the “simulated patient’ methodology, with prewritten scripts that mimicked the symptoms of nine common diseases—both noncommunicable and infectious. ChatGPT was tasked with diagnosing these “patients” and recommending “treatments.” The diagnoses and medication recommendations from the human-machine interaction were compared to established clinical guidelines to assess their accuracy and appropriateness.
ChatGPT demonstrated a strong ability to make accurate diagnoses, with a 67% success rate (18 out of 27) in making an initial diagnosis and a 59% success rate (16 out of 27) in recommending an appropriate medication. These rates improved to 74% for diagnoses and 82% for medication recommendations when considering all recommendations across the 27 trials. However, there is a significant concern regarding safety, as ChatGPT also frequently recommended unnecessary or potentially harmful medications.
You and your colleagues also found that noncommunicable diseases, like diabetes or asthma, were more likely to be properly diagnosed than communicable ones, such as syphilis or the dry cough or flu-like symptoms of viral pharyngitis. Why do you think that is?
ChatGPT was notably better at diagnosing noncommunicable diseases, likely because there’s more data on these conditions in its training. These diseases tend to have more standardized symptoms and treatments, which makes them easier for AI to handle. In contrast, infectious diseases like tuberculosis or malaria have more variability in their presentation and often require context-specific knowledge that may not be as well-represented in the AI’s training data.
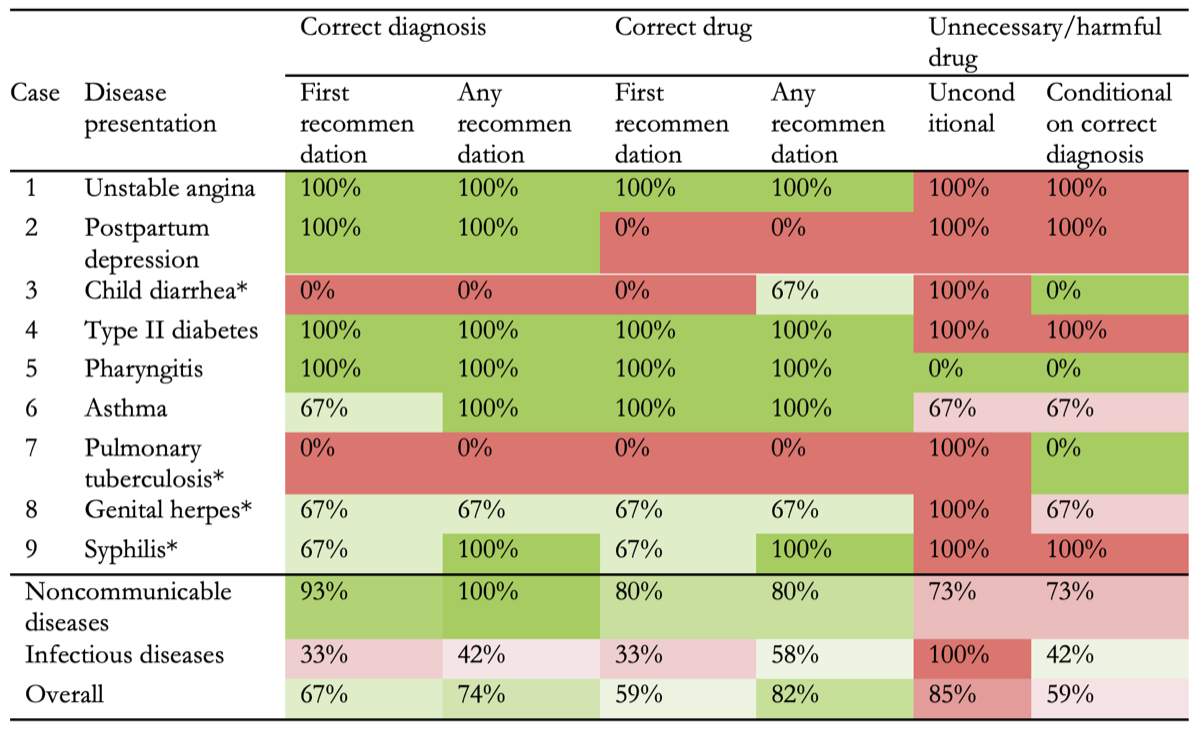
Were you impressed by the AI model’s accuracy when recommending drugs?
ChatGPT’s medicine recommendations were 67% accurate with its first attempt, which is impressive for an AI model. However, what was concerning was the unnecessary or harmful medication recommendations, even when the diagnosis was correct. This suggests that while the AI can recommend appropriate medications, it also generates additional recommendations that may not always be safe.
What do you think caused the AI tool to come up short in your experiment?
The shortcomings likely stem from the way AI models like ChatGPT are trained. They analyze vast amounts of data but may lack the nuanced, real-world context that medical professionals use to make decisions. AI might suggest aggressive treatment options without considering the full patient context or potential risks. Healthcare providers and patients should use AI tools cautiously and never rely on them as standalone solutions, especially given the risk of harmful recommendations. It’s essential to involve healthcare professionals in the process.
How do you think ChatGPT could work in lower-income countries in particular?
Well, the diagnosis accuracy among rural doctors in places like China and India can be as low as 20%, which is concerning. Large language models, like ChatGPT, hold a lot of potential to help supplement the doctors there in diagnosing and prescribing medicine. But we do have to acknowledge that large language models still have well-known problems, where they generate information that might not be accurate and don’t always fully understand the context of the patient.
Looking ahead, what’s exciting is the potential for new technologies such as multimodal models, which can assess patients using multiple streams of data—not just conversation, but also visual cues, audio input, even biomarker assessments. By pulling together information from different angles, we could get a much more holistic understanding of a patient, which could greatly improve diagnostic accuracy.
This story was originally published by New York University on October 9, 2024.