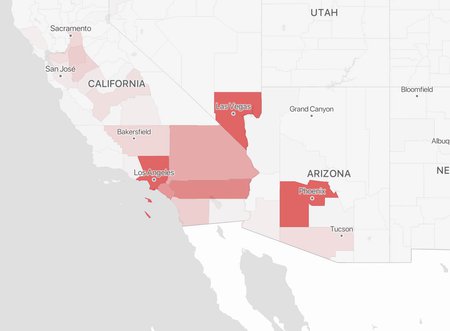
Researchers hope the model can inform public health decision-making amid the pandemic
A new model for predicting COVID-19's impact using artificial intelligence (AI) dramatically outperforms other models, so much so that it has attracted the interest of public health officials across the country.
While existing models to predict the spread of a disease already exist, few, if any, incorporate AI, which allows a model to make predictions based on observations of what is actually happening—for example, increasing cases among specific populations—as opposed to what the model's designers think will happen. With the use of AI, it is possible to discover patterns hidden in data that humans alone might not recognize.
"AI is a powerful tool, so it only makes sense to apply it to one of the most urgent problems the world faces," says Yaser Abu-Mostafa (PhD '83), professor of electrical engineering and computer science, who led the development of the new CS156 model (so-named for the Caltech computer science class where it got its start).
The researchers evaluate the accuracy of the model by comparing it to the predictions of an ensemble model built by the Centers for Disease Control and Prevention from 45 major models from universities and institutes across the country. Using 1,500 predictions as points of comparison with the CDC ensemble, the researchers found that the CS156 model was more accurate than the ensemble model 58 percent of the time as of November 25. It also routinely outperforms the benchmark projections of the Institute for Health Metrics and Evaluation (IHME).
The CS156 model is an amalgam of several models developed by Abu-Mostafa and his group over the past nine months; the weight that each model has on the overall CS156 model's output is determined based on its performance over the previous weeks (using statistics reported in The New York Times for comparison).
Abu-Mostafa is currently expanding the CS156 model based on feedback from public health officials in the hope that it can be a lifesaving tool to guide policy decisions. He has been contacted by officials from the California Department of Public Health (CDPH) and from representatives of the New York City Commissioner of Health.
Based on their feedback, the model is being modified to allow public health officials to predict how various interventions—like mask mandates and safer-at-home orders—affect control of the spread of the disease. Armed with those predictions, public health officials would be better able to evaluate which interventions are most likely to help. CDPH already uses the CS156 model in its decision-making, Abu-Mostafa has learned.
"We are working on this feverishly," says Abu-Mostafa, whose group has gathered data on every COVID-19 policy in every county in California since the pandemic began. "But it's a tricky problem. Demographics matter. Younger people tend not to abide by public health guidance as much as older people, and policies targeted toward businesses seem to be more effective than ones targeted toward individuals. But we're still digging into this."
The CS156 model began as a competition in Abu-Mostafa's computer science class, CS/CNS/EE 156, Learning Systems, during Caltech's spring 2020 term. The class normally would have applied AI to a much more benign topic, such as movie recommendations, but Abu-Mostafa and his students saw an opportunity to make a real difference with their work. Eighty students were already in the class; an additional 70 signed up when the new challenge was announced. By the end of the term, the class had generated 40 viable models, 10 of which were already competitive with existing epidemiological models.
Throughout the summer, Abu-Mostafa and a core group of students from the class continued the work, refining their data collection and modeling. They officially launched the CS156 model on August 24. This fall, when the students returned to their studies, Abu-Mostafa continued to manage the aggregation of the models, although he attributes the continued success of the CS156 model to the hard work of his student colleagues.
"At this point, I'm like a chef cooking a meal," Abu-Mostafa says. "You need good ingredients; without them, the meal cannot be good. But even with them, you still have to work hard to prepare the meal right."
Abu-Mostafa hopes that news of the efficacy of the CS156 model will bring it to the attention of additional public health policy makers, who can use it in their decision-making and potentially save lives.
"All of us who are working on COVID-19 models are working toward the same goal: winning the war against the pandemic. We're here to do our part," he says.
The student research that led to the creation of the CS156 model was supported by Caltech Trustee and alumnus Charles Trimble (BS '63, MS '64); the Summer Undergraduate Research Fellowship program; and the Clinard Innovation Fund, established by entrepreneur and Caltech alumnus by Gary Clinard (BS '65, MS '66).
This story was originally published by the California Institute of Technology.