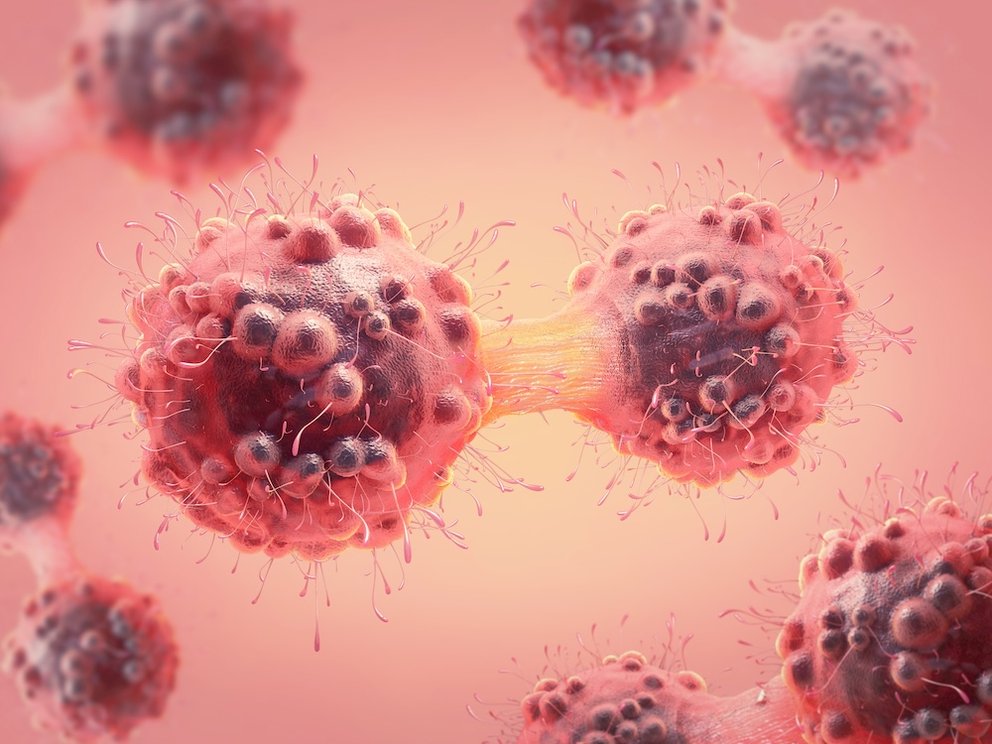
Stampede2, Lonestar6, Corral systems help advance tumor models, treatment options
Breast cancer leads worldwide among cancers in women, claiming nearly 670,000 lives in 2022 according to the World Health Organization. TACC supercomputers give scientists the computational resources and innovative data analysis tools they need to make new discoveries in understanding and treating breast cancer. The following examples illustrate different strategies where advanced computing is making strides in conquering breast cancer.
Digital Twins in Oncology
Mathematical modeling has helped improve predictions of how triple-negative breast cancer (TNBC) tumors will respond to treatment, according to research led by Tom Yankeelov of the Oden Institute for Computational Engineering and Sciences at UT Austin.
TNBC cells lack three commonly overexpressed biomarkers in breast cancer — receptors of estrogen, progesterone, and the human epidermal growth factor receptor 2 (HER2). TNBC is an aggressive form of breast cancer with fewer treatment options and is more common in Black women and all women under 40.
Yankeelov co-authored a 2022 study published in the journal Cancer Research that used MRI data from 56 patients with TNBC to develop calibrated models to achieve early, patient-specific resolved predictions of the tumor response of patients with TNBC.
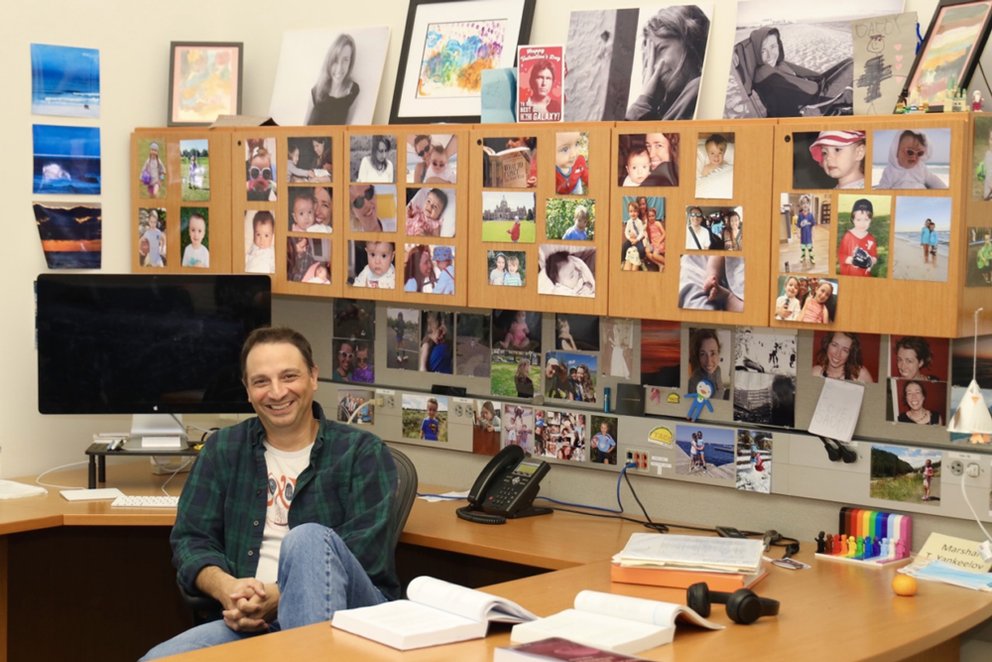
“Using patient specific imaging data, we calibrated our biology-based, mathematical model to make predictions of how tumors grow in space and time,” Yankeelov said. “These predictions have shown to be highly accurate when predicting the response of triple negative breast cancer patients to standard neoadjuvant chemotherapy.” This type of chemotherapy is widely accepted as the standard-of-care for early TNBC, but it comes with concerns of clinical benefits versus harm from the treatment.
Improved predictions provide physicians with guidance on whether a particular treatment is likely to work. “If our model predicts that the treatment is going to be beneficial, then they have more confidence staying the course with chemotherapy. Conversely, if our model predicts that the treatment is not going to be beneficial, then they have more confidence finding an alternative intervention,” Yankeelov said.
"Over the last eight years, TACC has provided extensive computational support for our research efforts via Lonestar5, Lonestar6, and Frontera. TACC has been there every step of the way as we develop methods for improving the treatment of, and outcomes for, patients battling cancer."
Yankeelov’s mathematical models describe how tumor cells change in space and time due to factors such as how the cells migrate, how they proliferate, and how they respond to therapy.
“What we do is make MRI measurements that let us calibrate those model parameters based on an individual patient's MRI measurements," Yankeelov said. "Once the model is calibrated, we run it forward to predict how that patient's tumor will grow in space and time —this prediction can then be compared to actual measurements in the patient at a future time. It is these predictions that we are getting surprisingly good at.”
Going forward, his lab’s goal is to go beyond making a prediction of whether a patient will respond to therapies or not. Instead, it is about using mathematical modeling to identify an optimal intervention strategy.
“If you have a model that can accurately predict the spatial and temporal development of a tumor, then we use a supercomputer to try an array of treatment schedules to identify the one that works best. That is, we use the mathematical model to build a 'digital twin' to try a myriad of treatment schedules to identify the one with the highest probability of success. That is where the research and field is going,” Yankeelov added.
Yankeelov’s lab used TACC’s Stampede2 and supercomputer and Corral high performance storage in developing digital twins. It's a fast turnaround — the goal is to get the digital twins to work within 24 hours of getting a patient's data to help a physician with treatment decisions within 24 hours, according to Yankeelov. To reach that goal requires access to a supercomputer.
"Over the last eight years, TACC has provided extensive computational support for our research efforts via Lonestar5, Lonestar6, and Frontera," Yankeelov said. "Indeed, it started within the first weeks of our arrival in Austin where TACC staff visited our lab to provide a rapid tutorial on how to start using the systems. TACC has been there every step of the way as we develop methods for improving the treatment of — and outcomes for — patients battling cancer."
HER2+ and Combined Therapies
HER2+ breast cancer overexpresses the gene that makes the HER2 protein —it is characterized as an aggressive breast cancer that can respond well to treatments such as Trastuzumab (a monoclonal antibody), which typically is administered in combination with Doxorubicin (a chemotherapy drug). The challenge for researchers and physicians lies in optimizing the combination of these two drugs to maximize treatment efficacy.
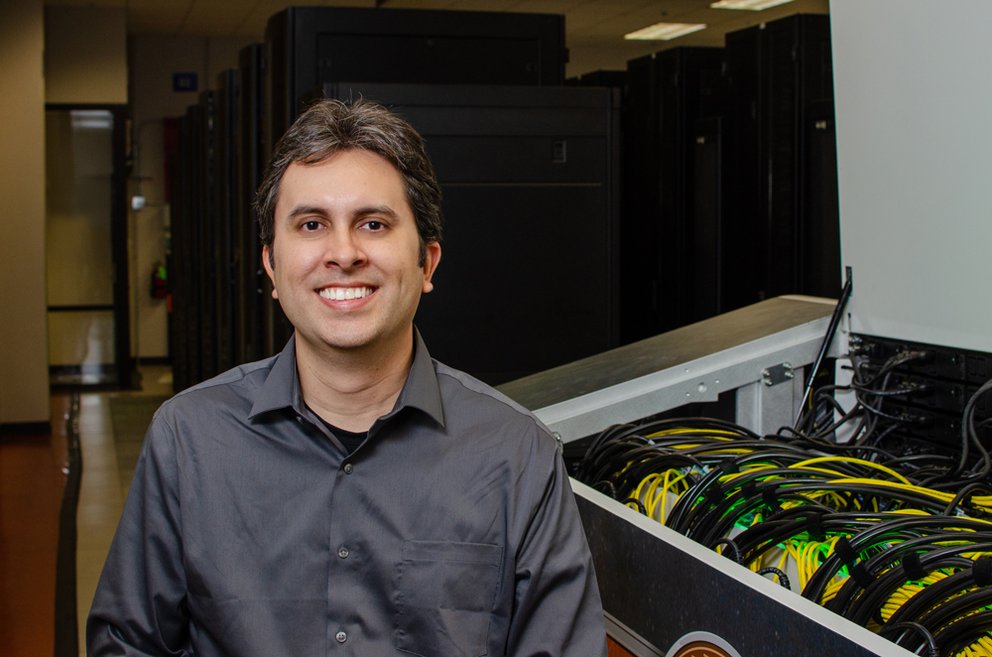
“I developed several mathematical models to assess their ability to replicate experimental data with mice receiving various drug combinations obtained by our collaborator Anna Sorace,” said Ernesto Lima of the Oden Institute.
Lima co-authored along with Yankeelov a 2022 study published in Computational Methods in Applied Mechanics and Engineering. It developed a family of models to capture the effects of combination Trastuzumab and Doxorubicin on tumor growth to optimize the outcome of the combination therapy while minimizing the dosage and thereby the toxic side-effects necessary to achieve tumor control.
“We created 10 models and calibrated them using the experimental data," Lima said. "Calibration involves adjusting parameters, such as the proliferation rate, which dictates how fast the tumor volume increases over time to align the model's output with the experimental data."
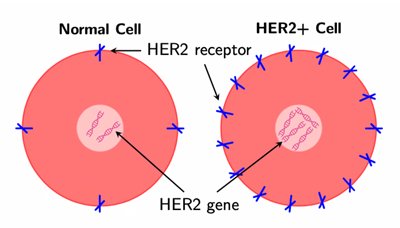
Lima was awarded supercomputer allocations through The University of Texas Research Cyberinfrastructure project on TACC’s Lonestar6 system to calibrate the models, computations that when parallelized ran 13 times faster than in serial. Parallelization takes large calculations and divides them into smaller ones that run simultaneously, versus running the calculations one-at-a-time.
After identifying the best model to replicate the data, Lima’s team optimized the treatment protocol.
“Using our model, we determined the optimal order and timing of drug delivery to maximize treatment efficacy. One treatment protocol, with the same drug amount as in the experiments, achieved a 45 percent reduction in tumor size compared to the experimental controls,” he said.
The team sought ways to maintain treatment efficacy while reducing the drug concentration because of potential toxicity. “We successfully reduced the concentration of Doxorubicin by almost 43 percent, while maintaining the same treatment outcome as in the experiments,” Lima added.
"Without TACC, our ability to explore diverse treatment options and solve complex mathematical models, driving forward our understanding of tumor biology, would be significantly hindered," he continued.
To validate their theoretical results, Sorace and her team are evaluating the identified protocols in a new set of experiments with mice. Preliminary results are hopeful — they suggest that the experimental protocol is more effective than the original protocols. However, there is a long road ahead before they can enter clinical trials.
“The experiments were done with a limited number of doses per drug and treatment protocols," Lima concluded. "However, the framework itself could be applied to different types of treatments where you have multiple drugs being delivered.”
Biopsy Data Gold Mine
UT Austin has gained a veritable goldmine of de-identified breast cancer data and preserved frozen tissue samples of other carcinomas, thanks to a generous donation in the spring of 2024 from James L. (Jim) Wittliff and his wife and collaborator, Mitzie, of the University of Louisville School of Medicine.
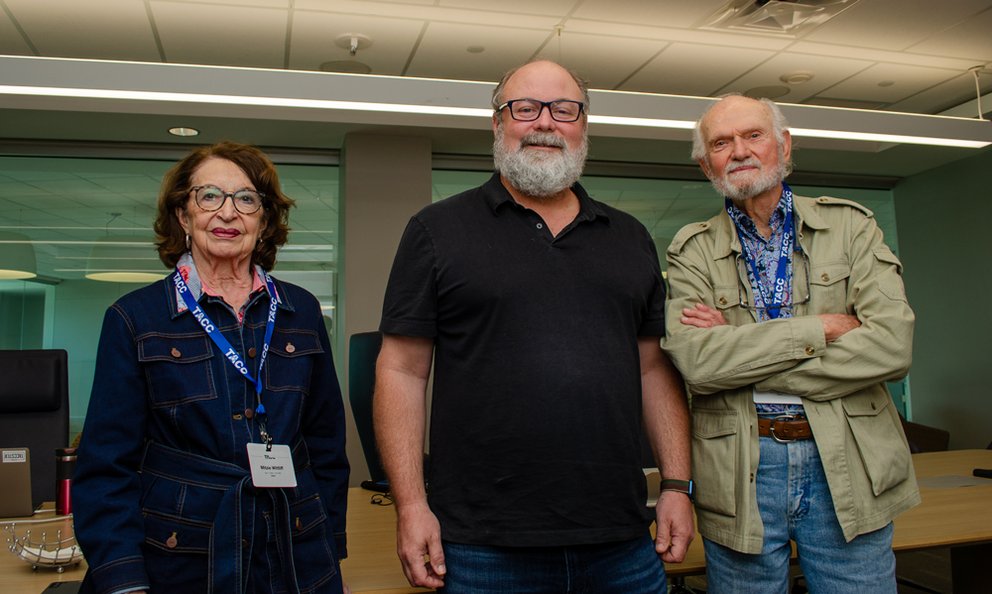
“This Database and Tissue Biorepository contains among the most highly quantified datasets of breast cancer biomarkers in the world, with several of the assays such as those for estrogen and progestin receptor proteins representing gold standard breast cancer tests," said Wittliff.
In the 1980s, Wittliff was co-developer with NEN/DuPont of these latter two biomarker tests which were approved by the FDA. More than 5,000 frozen pristine breast, endometrial, ovarian, and colon cancer biopsies and nuclear pellets containing DNA that were collected from patients that Wittliff’s Clinical Laboratory served and curated through a lifetime of research have been transferred and are now stored at the Dell Medical School. In addition, a treasure trove of de-identified comprehensive biomarker and clinical data will be stored and managed at TACC.
“Our immediate goal is to analyze these data, probably in the context of the NIH’s The Cancer Genome Atlas Program and other data," said Ari Kahn of the Life Sciences Computing Group at TACC.
"Wittliff is energized to expedite the use of the comprehensive data and unique samples to advance cancer diagnosis, treatment approaches, and ways to assess risk of recurrence of carcinomas, and is excited to support UT Austin, his alma mater, with this amazing gift. TACC will steward the data on TACC's Corral system and is planning on making it available in the future to other scientists online through tools such as a web portal." - - Ari Kahn, Life Sciences Computing Group, TACC
The irreplaceable biopsies are now preserved for other scientists to use for clinical trials in silico and to develop future companion diagnostic tests. Many of the tissue specimens have data associated with them such as protein tumor markers; genomic data on gene expression; patient characteristics such as age, sex, and smoking history; disease properties such as tumor size and pathology; and clinical follow-up such as surgeries and chemotherapy treatments.
“Wittliff is energized to expedite the use of the comprehensive data and unique samples to advance cancer diagnosis, treatment approaches, and ways to assess risk of recurrence of carcinomas, and is excited to support UT Austin, his alma mater, with this amazing gift,” Kahn added. “TACC will steward the data on TACC's Corral system and is planning on making it available in the future to other scientists online through tools such as a web portal."
Cancer and AI
Artificial intelligence has emerged as a tool for the sciences helping researchers make progress on biological problems such as high throughput virtual drug screening and planning chemical synthesis pathways. According to Yankeelov, it is important to point out the fundamental limitations of AI in what it can inform scientists about the most important problems in oncology.
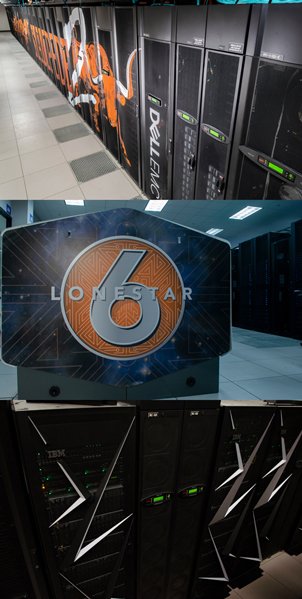
"In studying cancer, the problem with the AI approach is that cancer is a notoriously heterogeneous disease. In fact, it is not just one disease — it is more than 100 diseases. The issue is with needing a training set to calibrate an AI algorithm," Yankeelov said.
For example, consider a patient with TNBC cancer who contracts one of the five different subtypes of triple-negative breast cancer that are labeled.
“To use an AI-based approach to predict how this patient needs to be treated, one needs to have a training data set that consists of that subtype of triple-negative breast cancer in addition to all of the possible therapeutic regimens that could be received," Yankeelov said.
"That training set does not exist, and it will never exist because the diseases are getting more specifically labelled and the treatments are getting more targeted. Furthermore, even if it did exist, it does not account for the unique characteristics of this patient's cancer because the patient is different than everyone else in that training set."
Challenging Road Ahead
Cancer remains one of the biggest health challenges facing society. According to Yankeelov and Lima, the computational resources provided by TACC are essential in advancing tumor models and treatment options by facilitating rigorous testing and refinement of various mathematical models.
TACC offers scientists the computational resources they need to make discoveries that are effective for breast cancer patients. Rising survival rates over the past decade for breast cancer offer a glimmer of hope, thanks to awareness campaigns and increased funding for research.
This story was originally published by the University of Texas at Austin on May 20, 2024.